欧洲科学院院士、IEEE Fellow王子栋教授系列课程通知
西交•通全球暑期学校(XJTISS)
欧洲科学院院士、IEEE Fellow王子栋教授系列课程通知
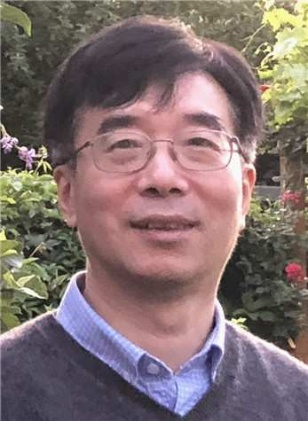
个人简介:Zidong Wang,现任英国伦敦Brunel University讲席教授,欧洲科学院院士,欧洲科学与艺术院院士,IEEE Fellow,International Journal of Systems Science主编,Neurocomputing主编。多年来从事控制理论、机器学习、生物信息学等方面研究,在SCI刊物上发表国际论文七百余篇。现任或曾任十二种国际刊物的主编、副编辑或编委。曾任旅英华人自动化及计算机协会主席、东华大学国家级领军人才、清华大学国家级专家。
Personal Profile:
Zidong Wang is currently a Chair Professor at Brunel University London, a Fellow of the European Academy of Sciences, a Fellow of the European Academy of Sciences and Arts, an IEEE Fellow, and the Editor-in-Chief of the International Journal of Systems Science and Neurocomputing. He has been engaged in research on control theory, machine learning, bioinformatics, and other fields for many years, and has published over 700 international papers in SCI journals. He is currently or has previously served as the Editor-in-Chief, Associate Editor, or Editorial Board Member of twelve international journals. He has also served as the President of the Chinese Automation and Computing Society in the UK, a National Leading Talent at Donghua University, and a National Expert at Tsinghua University.
方式1:线下
地点:巨构2号楼2-2072
方式2:线上
地点:腾讯会议号865 786 333
课程题目:Deep Learning in Industry Applications
课程简介:This short course is to provide an in-depth introduction to the area of learning using deep neural networks by presenting a wide variety of deep neural network architectures and their learning methods, including deep fully-connected networks, convolutional networks, and generative adversarial networks etc. The main focus of the module is to develop student's skills in analysing problem requirements, applying appropriate deep learning methods to various real-world problems, and evaluating the effectiveness of the adopted methods.
面向对象:高年级本科生及研究生
具体时间安排:
日期 |
9:00-10:00 |
10:20:11:20 |
14:30-15:30 |
15:50-16:50 |
7月28日 |
课时1 |
课时2 |
课时3 |
课时4 |
7月29日 |
课时5 |
课时6 |
课时7 |
课时8 |
7月30日 |
课时9 |
课时10 |
|
|
具体章节介绍:
Lecture Topics
1.Introduction to Deep Learning
2.Machine Learning: A Quick Review
3.Neural Networks I
4.Neural Networks II
5.Deep Neural Networks
6.Convolutional Neural Networks I
7.Convolutional Neural Networks II
8.Generative Adversarial Networks I
9.Generative Adversarial Networks II
10.Big Data Analysis at Brunel University
“西交-通全球暑假复合材料课程”微信群&腾讯会议线上链接